Methods
We study human perception and decision-making. We base our studies on experimental approaches from perception research (psychophysics in general and signal detection in particular), behavioural economics and neuroimaging. We mostly test healthy participants, and sometimes patients or participants who have received a pharmacological compound. The data we collect are behavioural choices, eye position data and magnetic resonance imaging data. Below are details on a few key methods that we employ in many of our studies.
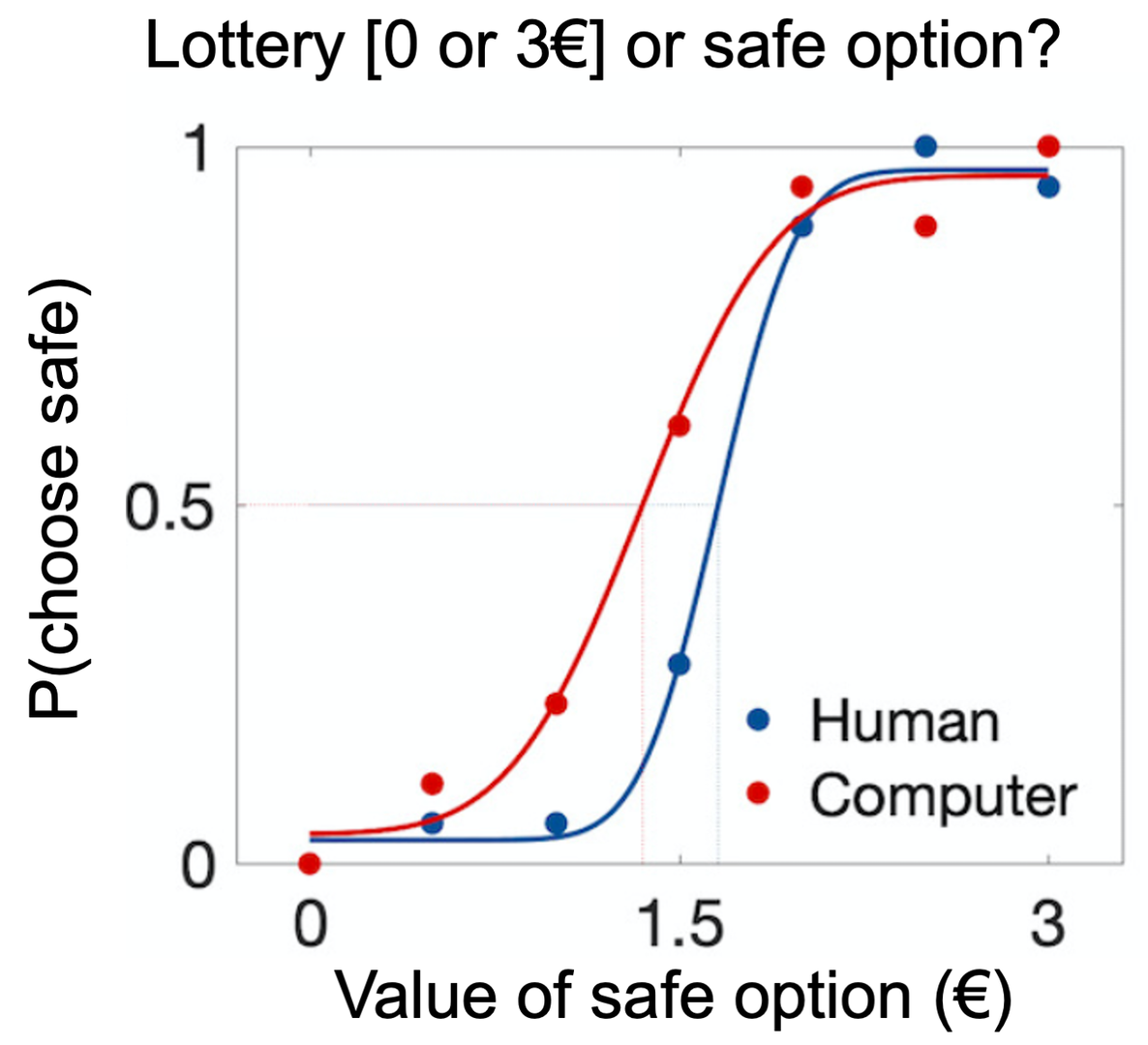
Experimental paradigms
We use methods from psychophysics and behavioural economics to assess how people form decisions based on stimuli and choice options. Whenever possible, we use parametric tasks and binary responses to relate gradual changes in the stimuli and choice options to choices through functions that represent assumed neural computations. The image shows results of a value-based decision task, in which participants are asked whether they prefer a lottery (0 or 3€, equiprobable outcomes) or a safe monetary outcome (varied across trials). Participants witness the outcome of their choice, paired with social or non-social feedback when the lottery was chosen. Participants with low levels of social anxiety choose the lottery more often when it includes social feedback. See Schultz et al., eLife 2019 for details.
BOLD signal as a measure of neural activity
We use magnetic resonance imaging (see top of image) to measure blood-oxygen-level-dependent (BOLD) signals, which are haemodynamic changes that occur after increases in neural activity. The lower part of the image shows results of a classic study relating neural activity and BOLD (Logothetis et al., Nature 2001). LFP is the local electric field potential, MUA is the multi-unit activity, and SDF is the spike-density function, which represents a neuron's instantaneous firing rate. Localised BOLD signal changes allow us to infer which neural structures are involved in the processes we study.
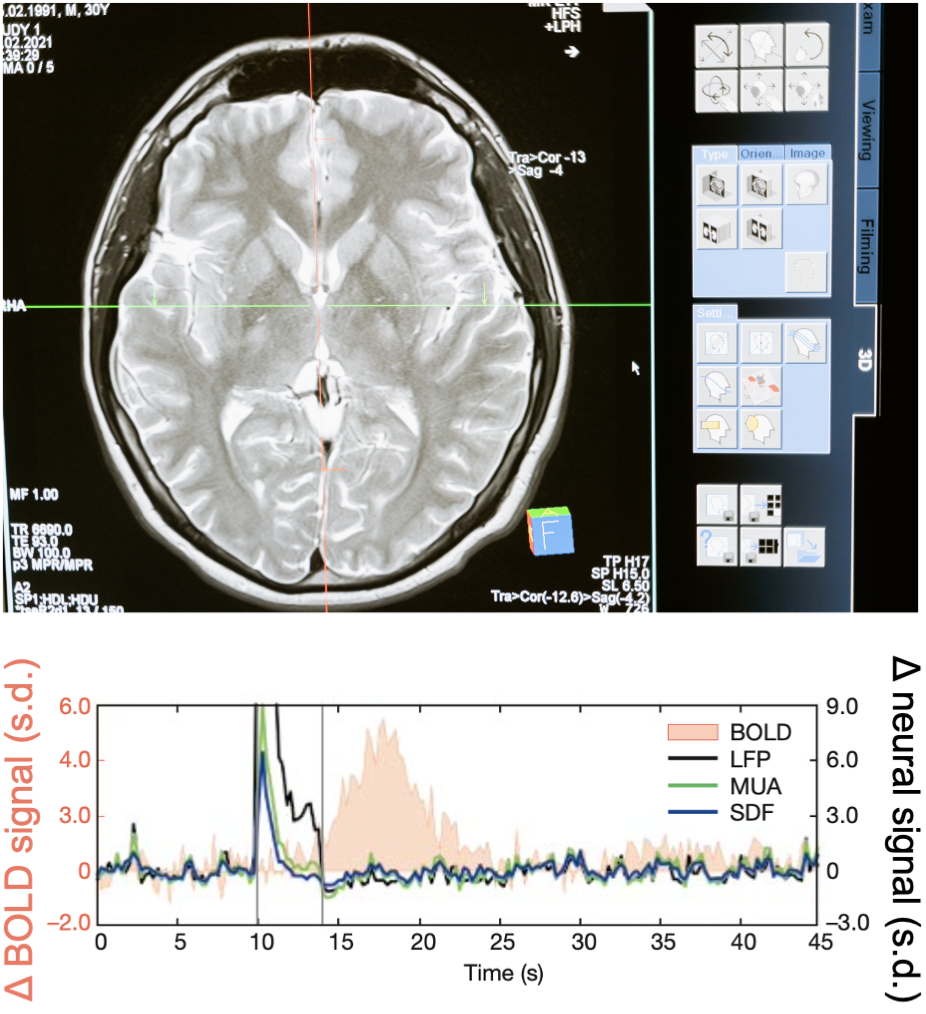
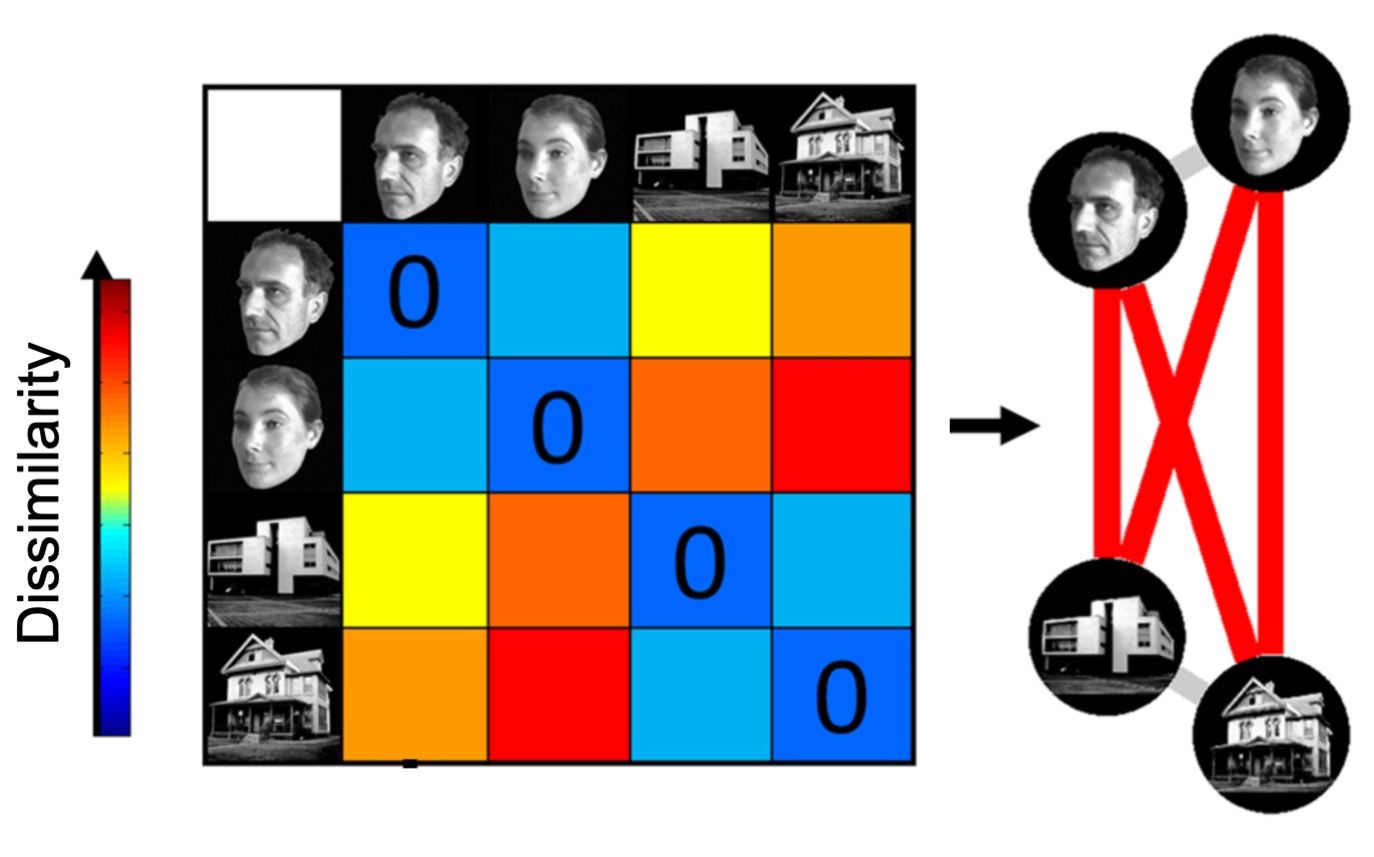
Estimates of neural representations
BOLD signal changes are typically analysed by fitting data time-series with general linear models (GLM), resulting in parameter estimates maps. Classically, these maps are then compared across experimental conditions using (mass-)univariate statistics. Increasingly popular multivariate analysis methods allow to use patterns of response estimates as features to train machine learning algorithms or as input for representational similarity analyses (see the image for an example). This allows to estimate neural representations from BOLD signal, and compare these to other representations.
Replication and preregistration
Nobody really benefits from irreproducible results. One approach we use to improve the robustness of our findings is by including ways to replicate previous findings by ourselves and others in our experimental designs. The figure shows 3 replications of a response to natural face motion in the right lateral temporal cortex, all p<0.05 FWE-corrected. For untested paradigms, we use discovery – validation designs (for example, here). We try to preregister our studies or analyses. We increasingly make our experiment code, data and analysis code publicly accessible.
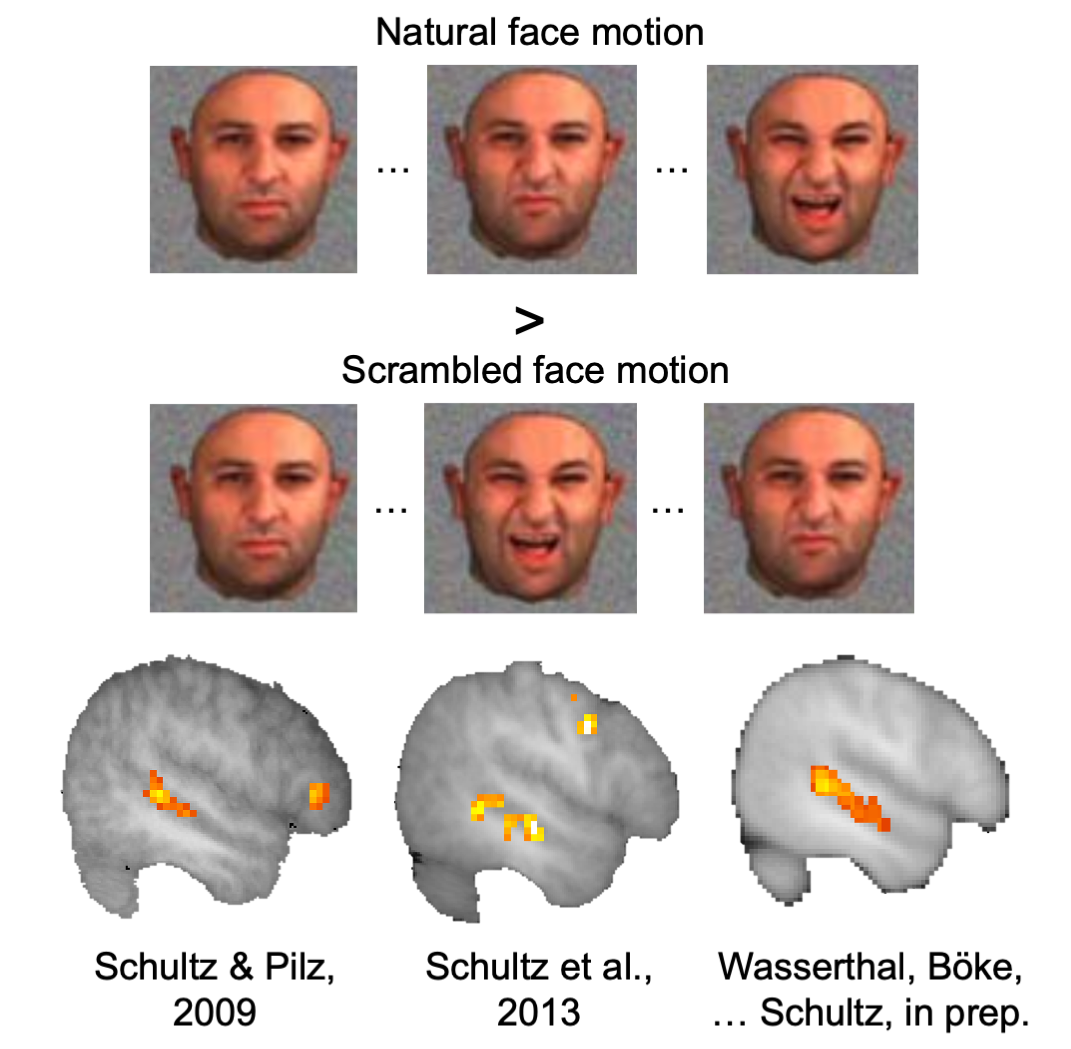